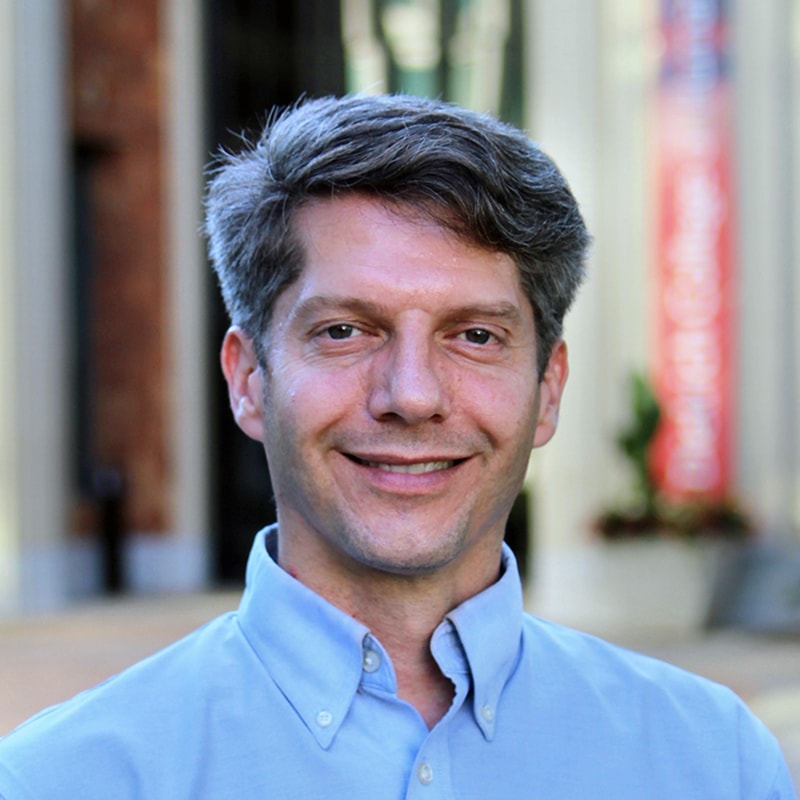
Alex John London
Clara L. West Professor of Ethics and Philosophy
In this course, we explore the concept of responsible artificial intelligence, misconceptions surrounding it, and its recent rapid growth and development. We will discuss the benefits and potential applications of responsible AI and the ethical and social concerns surrounding its use. We will also discuss the importance of ensuring that AI is developed and used in a way that is fair, transparent and accountable.
This seven-week, online, self-paced course complements online content with live weekly office hours and live Q&A sessions with learning facilitators.
In each module, we define a specific concept in the context of responsible AI systems, and discuss its benefits and limitations. We discuss how to account for this factor as part of responsible AI development, important considerations and common misconceptions for each, and recent developments in this space.
This course has been designed for leaders, managers and executives who work on AI products or with AI product teams. The course does not assume any background knowledge of AI and there are no technical prerequisites.
We recommend a cohort size of 30 students. Custom cohorts can be launched with short notice.
Upon completion of the module, participants will be able to:
Upon completion of the module, participants will be able to:
Upon completion of the module, participants will be able to:
Upon completion of the module, participants will be able to:
Upon completion of the module, participants will be able to:
Upon completion of the module, participants will be able to: